Norway boasts over 100,000 km of coastline, including islands and reefs, with only a fraction of its species and habitats mapped in detail. In these regions, there are endangered species and vital ecological components, such as eel grass, seaweed, and kelp, which serve as carbon stores, nursery areas, and food sources for diverse coastal ecosystems.
Now, drones are revolutionising the mapping and monitoring of Norway's marine nature, especially in this challenging coastal zone where traditional methods face limitations.
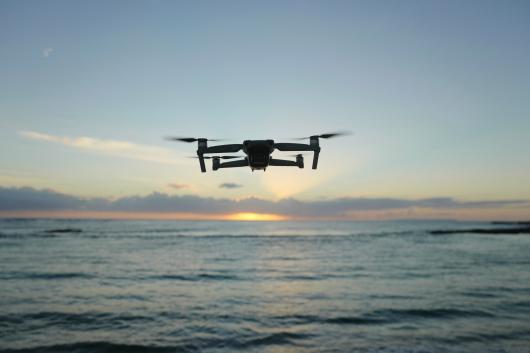
Classifications by AI
The SeaBee project led by NIVA (Norsk institutt for vannforskning) provides a research infrastructure to help Norwegian scientists map and monitor the coastal zone faster and more efficiently than what can be done by plane or boat. SeaBee pilots use flying drones and other autonomous vehicles to collect high-resolution imagery of coastal areas. State-of-the-art machine learning algorithms are then used to automatically classify the images – for example by counting seabird and seal species, or by mapping shallow-water habitats (e.g., eelgrass beds). The results are used to support research and inform coastal management.
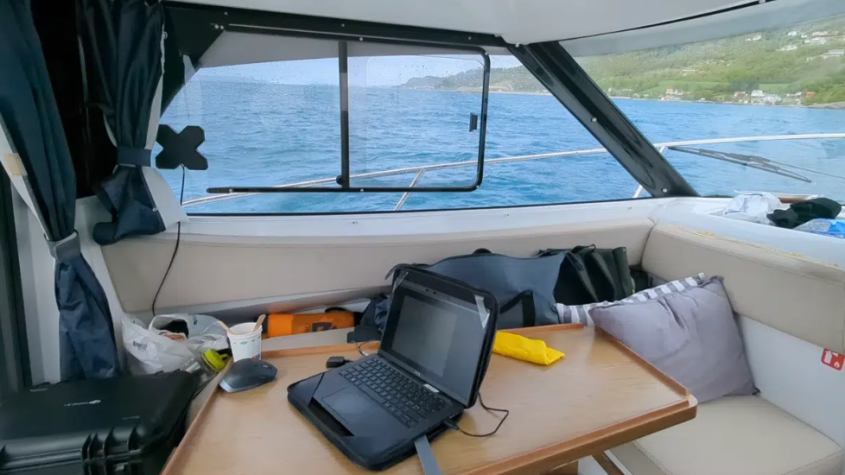
— During this time, we have received fantastic support from Sigma2, allowing us to scale SeaBee workflows far beyond what we could achieve using “on premise” hardware, says James Edward Sample, Senior Engineer at NIVA.
Raw images from drone missions are uploaded directly to the SeaBee data pipeline, which is hosted on the National Infrastructure for Research Data (NIRD), then processed using state-of-the-art ML algorithms, high-performance storage, and graphics processing units (GPUs) on the NIRD Service Platform. New datasets are detected automatically and processed to create detailed mosaic images, which are then passed to the machine learning algorithms and published online. During 2023, the SeaBee platform running on NIRD processed data from over 600 drone missions generating more than 25 TB of data.
Open science and storage optimisaton
SeaBee plans to share data openly and free of charge, using Sigma2`s cost-efficient, long-term data storage. Therefore, the SeaBee project should find the next generation NIRD Research Data Archive which is underway to be a good solution for their archiving needs, supporting the Open Science model. The ability to efficiently archive “secondary” datasets (such as point clouds or texture models) will be valuable, allowing Sample and his colleagues to reduce costs and conserve “high availability” storage for key data products. The new Research Data Archive will of course also support publishing of finished datasets with easily citeable DOIs, which are essential for reports and scientific publications.
SeaBee researchers are currently working towards a SeaBee2 project, which aims to expand the SeaBee infrastructure to take advantage of both the latest drone technology and recent rapid advances in AI.
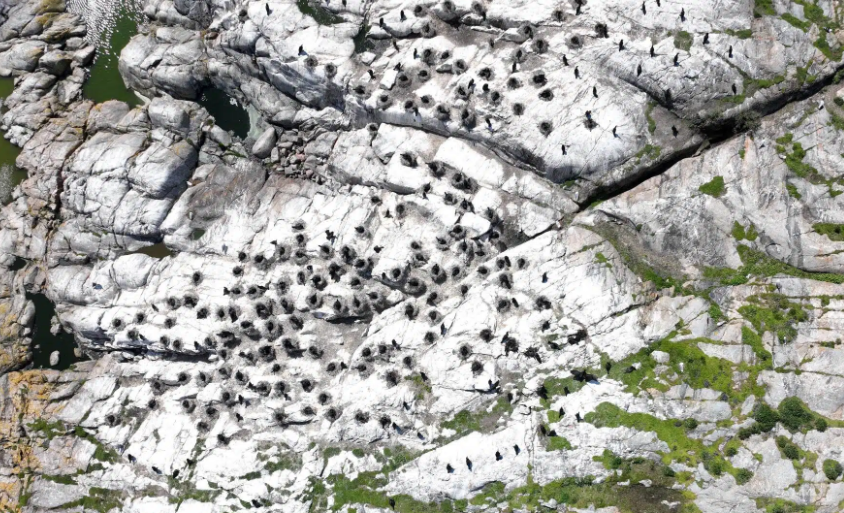
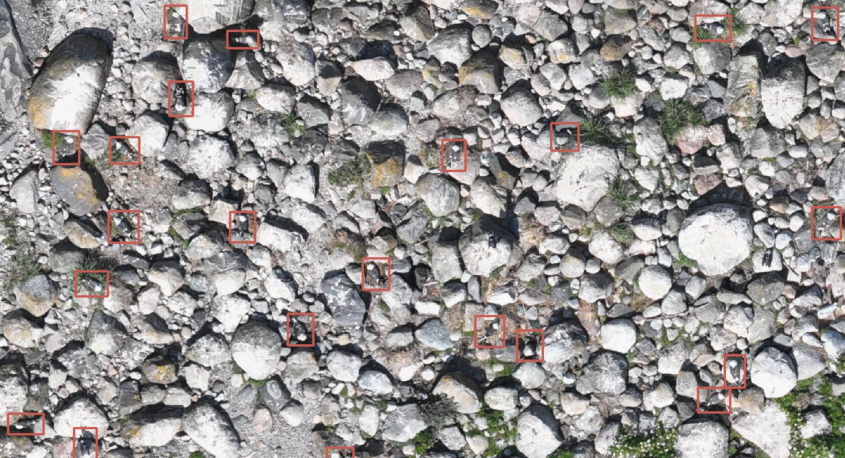
— SeaBee2 hopes to upscale and improve SeaBee workflows, allowing us to fly larger missions, faster and at higher resolution than ever before. For this, the new NIRD Research Data Archive will become a key component of the SeaBee infrastructure. We are very excited about SeaBee2 and, if successful, look forward to further collaboration with Sigma2.