Alarm systems play a vital role in ensuring safety and reliability in the process industry. In a process plant environment, operators are notified by alarms if a process diverges from normal operating conditions.
Alarms are triggered to warn the operator about irregular incidents. When several alarms go off simultaneously it is known as “alarm floods”, and the operator may miss the crucial alarms. Ideally, an alarm should therefore inform the operator about critical conditions only and provide guidance to a set of corrective actions associated with each alarm.
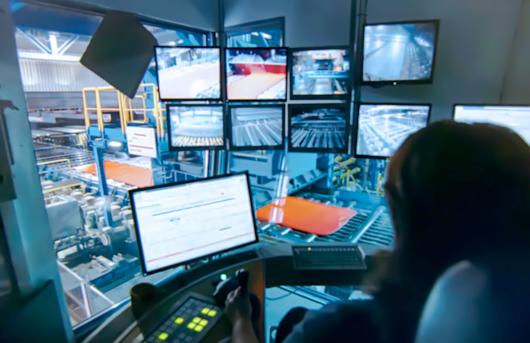
Advanced User Support Boosts Research at NTNU
Associate Professor Nicola Paltrinieri and his colleagues at the Department of Mechanical and Industrial Engineering at NTNU have benefited from an Advanced User Support (AUS) project. This project led to the publication of three scientific articles based on research conducted through the NIRD Service Platform and the Deep Learning Toolkit. The AUS project played a crucial role in the development of a new method for assessing dynamic chattering alarms, which was used to train and evaluate a Deep Neural Network. Subsequently, the model's performance was tested for its ability to predict alarm chatter
Enhancing Alarm Chatter Prediction with Run Length-Based Analysis
A modified approach, based on the distribution of run lengths, has been developed to assess the likelihood of future alarm chatter. This method enables the categorisation of historical alarm events as either prone to future chatter or not. Subsequently, these categorised alarms have been utilised to train a Deep Neural Network using TensorFlow. The performance of this network has been evaluated for its ability to predict alarm chatter.