Iris.ai is a Norwegian-founded company developing AI-powered tools to help researchers and businesses navigate the ever-growing scientific literature and technical documents.
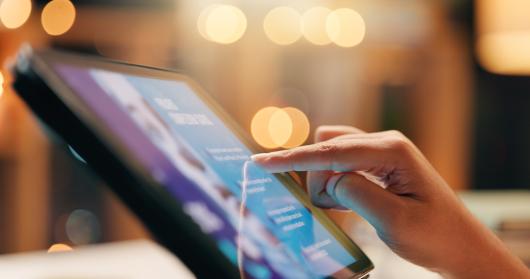
Designed for scientific text
"The capacity offered by Sigma2 allows us to train and develop more resource-intensive models from scratch, which would otherwise be unobtainable right now. This initiative will greatly support us in building services that help researchers innovate faster".
Anita Schjøll Abildgaard, CEO of Iris.ai.
The Iris.ai project focuses on building a Machine Learning (ML) system for scalable domain adaptation of Language Models (LLMs) designed specifically for scientific text.
Given the vast amount of new scientific knowledge produced daily, researchers struggle to keep up. To address this challenge, Iris.ai has developed the Researcher Workspace (RSpace)—a platform designed to efficiently process and interact with scientific knowledge.
The RSpace platform integrates:
- Content-based search with AI-driven keyword recognition
- Smart filtering to refine relevant results
- Machine-generated summaries for quick information retrieval
- Automated data extraction for structured insights
- Interactive tools that allow researchers to explore information dynamically
Key features
Iris.ai offers several core functionalities to streamline research workflows, including:
- Automated literature search: AI models analyse key concepts and find relevant scientific articles.
- Knowledge mapping: Creates visual representations of research fields based on article relationships.
- Document summarisation: Extracts the essence of scientific texts into concise summaries.
- Text-to-text Machine Learning: Assists in rephrasing and understanding complex research papers.
To develop and train the advanced machine learning models underpinning RSpace, Iris.ai requires significant computational resources. The complexity of their AI-driven research necessitates access to the national HPC resources for:
- AI-driven scientific research
- Training and fine-tuning LLMs for processing vast amounts of scientific literature.
- Semantic understanding to interpret and extract insights from complex research texts.
- Data synthesis to generate concise summaries, research overviews, and hypothesis suggestions.
- Generative AI for enhanced research
- Model training: Developing resource-intensive models for text generation, literature reviews, and knowledge discovery.
- Advanced querying: Enabling researchers to interact with large datasets through AI-assisted conversational search.
- Real-time monitoring and analysis
- Dynamic updates: Automatically tracking new publications, patents, and datasets.
- Automated curation: Filtering and structuring data into actionable insights.
Access to supercomputers Saga and LUMI
By employing the national HPC infrastructure, Iris.ai accessed on-demand computing power without acquiring expensive specialised hardware. By providing scalable and efficient HPC access, aided by the National Competence Center for HPC which was established to ensure that organisations like Iris.ai get the support and resources they need for research and development.
Enabling organisations like Iris.ai with computational resources levels the playing field in AI research and development. We are excited to witness that our infrastructure supports innovation at SMEs and startups, often the birthplace of groundbreaking ideas, who may lack the hardware capacity and expertise to enable their ambitions.