What is commonly called "deep learning” has become the dominant paradigm in much contemporary machine learning research and development.
Deep learning emphasizes on training the computer to learn by experience to perform tasks such as self-driving cars, facial recognition, or natural language processing.
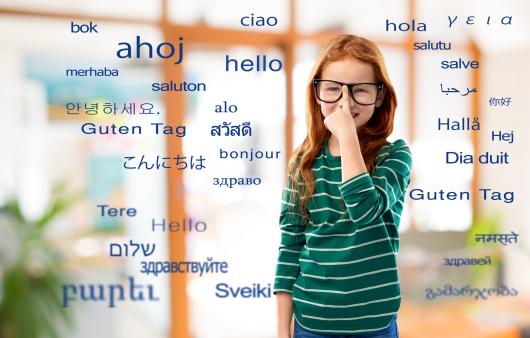
Fuelled by massive neural language models
In Natural Language Processing (NLP) breakthrough advances in recent years have been fuelled by massive neural language models, the best-known instance is BERT (Bidirectional Encoder Representation from Transformers), introduced by Google, LLC in 2018. These models are computationally expensive to train and refine, and user training can take up to several GPU months. Fine-tuning a pertained model for a specific application typically requires at least several GPU days. The models are currently only available for English and a few additional languages.
Limited GPU capacity
Mature and open-source deep learning frameworks like TensorFlow and PyTorch (by Google and Facebook respectively) in principle allow researchers without in-depth specialist training to conduct large-scale deep learning experiments that effectively parallelize across multiple GPUs or even multiple multi-GPU nodes. At present, there is only very limited GPU capacity available for research usage in Norway.
Professor Stephan Oepen and his colleagues at the Department for Informatics at UiO should be able to utilise the powerful LUMI supercomputer, of which Norway owns a part through Sigma2.